The power of personalisation and tailoring to the right audience
How machine learning best practices can support personalised e-commerce strategies that convert
Through its multiple outlets, our client – a global leisure organisation – had amassed a large store of customer data from multiple sources. The marketing team wanted to leverage data to improve communication with customers and increase sales. However, the quality of their data, a potentially rich source of information, was poor, with inconsistencies and low utility, limiting its accessibility to the business and therefore hindering its commercial potential. For example, inconsistent formats due to the disparate data management systems at multiple venues meant the business overall had problems with missing data and duplications which complicated data-based decision making.
Equal Experts investigated if machine learning techniques could predict which visitors would be most likely to buy memberships (the organisation’s most valuable source of income) so that marketing promotions could be personalised and targeted specifically to that customer segment. Creating a bespoke data product, the team then ran a trial which demonstrated the potential for a £1.6m uplift in sales by targeting the segment of high propensity customers identified by the model.
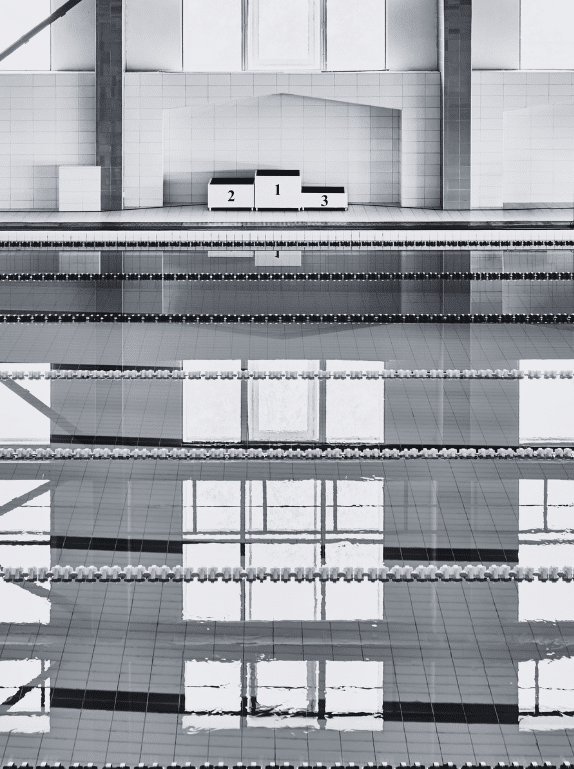
Outcomes
potential sales increase identified
increase from control group to target group
of purchases were made by people didn’t own a membership previously
About the client
Our client is a global leisure operator with multiple venues and income streams. As a leader in branded lifestyle outlets, it delivers high quality leisure experience to over 60 million guests every year.
Challenge
Transforming data into growth strategies
Our client operates multiple customer venues across the world. Each outlet operates as a business in its own right and collects rich data from customer sales. However, the business was not able to consolidate data from the different venues in a format that could help drive sales and inform business decisions. For example, visits to multiple venues by the same customer couldn’t be tracked to identify patterns of behaviour, so little was known about the likelihood of future purchases.
We thought there was an opportunity to improve the quality of data stores and use machine learning to predict where personalised marketing might have the most impact on membership sales across the group.
Solution
Making data work smarter, not harder
First, we cleaned up the data, processing millions of recent purchases to standardise data in a new data product. These processes, underpinned by a random forest machine learning model, formed our bespoke data product for the client. The new product serves as a foundation for understanding every guest who visits any of our client’s venues, and its data pipeline securely handles customer information from recent sales data. It can be used to derive insights about customers which can be used for personalised communications. These insights include:
- Travel distance to venues
- Active vs inactive customers
- Existing member renewals vs new purchases
Lifetime value of customers
The product is also used as the input into our machine learning modeling that predicts which guests would be most likely to buy a new membership. The model was able to predict which guests would be most likely to purchase an annual membership in the 2 weeks after a visit, to enable personalised marketing communications to be delivered to the highest propensity customers.
We ran a trial targeting a small percentage of the millions of guests attracted annually to the client’s venues. These were the customers who the model predicted represented a high chance of making a membership purchase (around 9,000 people). Here’s what we found in the test:
- 46% of customers who received an email within 2 weeks of visiting a venue responded
- Each guest would buy on average 2 memberships, which equated to potential sales of £1.6 million
Results
How targeted personalisation increased conversion
Results in the target group highlighted the following opportunities:
- 4.6% of targeted guests bought a membership, compared to 2.8% of guests from the control group
- 86% of purchases were for new memberships (not renewals)
- 74% of purchases were made by people who had never owned a membership previously
Even more significantly, when we narrowed our focus to target the most recent guests (from just the previous 2 weeks), nearly 50% responded to emails. The strong early sales suggest that our client could use this approach to personalise marketing throughout the year. By targeting high propensity guests, the model could help to generate in excess of £1 million per year for new memberships alone in the UK. There is potential to earn even more if the team targets existing membership customers for renewals, expands the initiative to venues outside the UK, and adds other ticket formats, hotels and value experiences to the model.
By making incremental improvements on the pipeline, automating testing and expanding the scope of data available to business users, the data product could drive significant sales over the long term for our client.
Our team demonstrated that properly structured data, combined with predictive modelling, can readily identify high-value opportunities with remarkable precision. This work showcases how the right data architecture and analytics can turn underutilised customer data into tangible business value.